CENTRA projects are developed through the rich discussions and interactions at CENTRA meetings. The collaborative projects combine the expertise, ideas, and experiences of our international community of scientists, educators, policy makers, and citizens.
New and Updated Projects
Other Projects
-
- AirBox
- AUAV Applications for Smart Agriculture
- Collaborative Lifemapper
- Dynamically Aggregating Smart Community Sensors, Edge and Cloud Resources with Overlay VPNs
- IT for Natural Disaster Management
- Efficient and Secure Data Management for HPC and Cloud Computing
- Persistent Identifier (PID) Kernel Information
- SDN-IP Peering for IoT Data Transmission
- The Visualization Alliance
AUAV Applications for Smart Agriculture (Updated)
Key Topics: Autonomous, UAV, Machine Learning, Agriculture
Start Date: December 12, 2018 (Updated: January 28, 2025)
End Date: April 30, 2026
The “AUAV applications for smart agriculture” project considers the use of unmanned aerial vehicle (UAV) for agriculture applications with integrating two major research directions together, edge computing for image processing with object recognition and autonomous UAV technique development. In the first joint publication, we focused on solving a real-world agricultural problem of rice lodging. Specifically, adaptive autonomous UAV (AUAV) scouting was proposed using edge computing and deep learning with EDANet for rice lodging assessments. Adaptive AUAV scouting includes different flying heights in one mission, thus, it can optimize cost and accuracy efficiently for practical lodging assessments. Using the proposed method, we can scout rice fields 36% faster than conventional scouting methods at 99.25% accuracy. For our future potential research, we would like to pursue UAV applications for Precision Agriculture (PA) such as identify crop damages, testing other algorithms for edge computing, testing ideas for AUAV functionalities.
Publication: Yang, M. D., Boubin, J. G., Tsai, H. P., Tseng, H. H., Hsu, Y. C., & Stewart, C. C. (2020). Adaptive autonomous UAV scouting for rice lodging assessment using edge computing with deep learning EDANet. Computers and Electronics in Agriculture, 179, 105817. (SCIE, IF8.3, 1/58 Agriculture category). https://doi.org/10.1016/j.compag.2020.105817.
CENTRA Primary Investigators: Ming-Der Yang, Director, National Chung Hsing University, Christopher Stewart, Co-director, Ohio State University, Hui Ping Tsai, Co-director, National Chung Hsing University, Jayson Boubin, Binghamton University, Cloud Tseng, National Chung Hsing University, Fang-Pang Lin and Hsiu-Mei Chou, NCHC
Project participants include: National Chung Hsing University (Taiwan): Ming-Der Yang, Director, Hui Ping Tsai, Co-director, Cloud Tseng; Ohio State University (USA): Christopher Stewart, Co-director; Binghamton University (USA): Jayson Boubin; NCHC (Taiwan): Fang-Pang Lin and Hsiu-Mei Chou
Start Date: December 12, 2018 (Updated: January 28, 2025)
End Date: April 30, 2026
The “AUAV applications for smart agriculture” project considers the use of unmanned aerial vehicle (UAV) for agriculture applications with integrating two major research directions together, edge computing for image processing with object recognition and autonomous UAV technique development. In the first joint publication, we focused on solving a real-world agricultural problem of rice lodging. Specifically, adaptive autonomous UAV (AUAV) scouting was proposed using edge computing and deep learning with EDANet for rice lodging assessments. Adaptive AUAV scouting includes different flying heights in one mission, thus, it can optimize cost and accuracy efficiently for practical lodging assessments. Using the proposed method, we can scout rice fields 36% faster than conventional scouting methods at 99.25% accuracy. For our future potential research, we would like to pursue UAV applications for Precision Agriculture (PA) such as identify crop damages, testing other algorithms for edge computing, testing ideas for AUAV functionalities.
Publication: Yang, M. D., Boubin, J. G., Tsai, H. P., Tseng, H. H., Hsu, Y. C., & Stewart, C. C. (2020). Adaptive autonomous UAV scouting for rice lodging assessment using edge computing with deep learning EDANet. Computers and Electronics in Agriculture, 179, 105817. (SCIE, IF8.3, 1/58 Agriculture category). https://doi.org/10.1016/j.compag.2020.105817.
CENTRA Primary Investigators: Ming-Der Yang, Director, National Chung Hsing University, Christopher Stewart, Co-director, Ohio State University, Hui Ping Tsai, Co-director, National Chung Hsing University, Jayson Boubin, Binghamton University, Cloud Tseng, National Chung Hsing University, Fang-Pang Lin and Hsiu-Mei Chou, NCHC
Project participants include: National Chung Hsing University (Taiwan): Ming-Der Yang, Director, Hui Ping Tsai, Co-director, Cloud Tseng; Ohio State University (USA): Christopher Stewart, Co-director; Binghamton University (USA): Jayson Boubin; NCHC (Taiwan): Fang-Pang Lin and Hsiu-Mei Chou
Contact: Hui Ping Tsai and Christopher Stewart
Email: huiping.tsai (at) gmail (dot) com, cstewart (at) cse (dot) ohio-state (dot) edu
Email: huiping.tsai (at) gmail (dot) com, cstewart (at) cse (dot) ohio-state (dot) edu
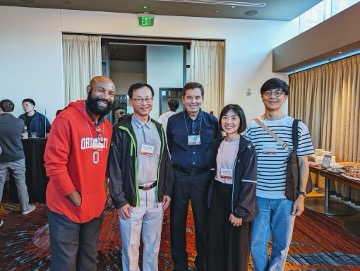
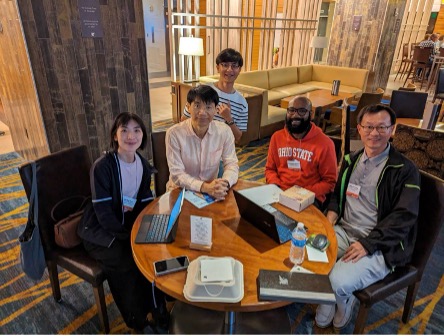
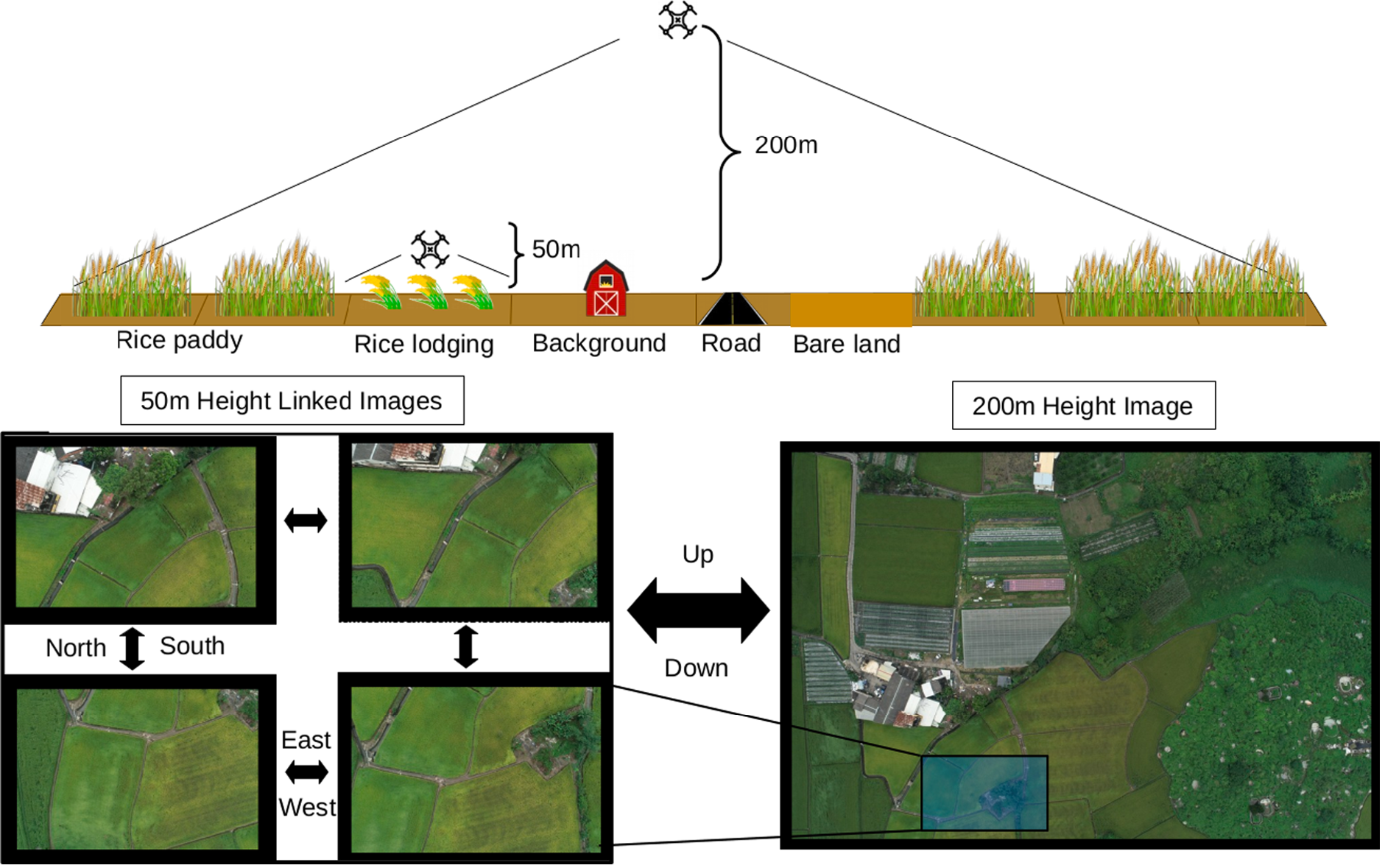
Federated Learning Framework for International Collaboration – using Scheduler Optimization of Computing Facility as Example
Key Topics: Federated Learning, Machine Learning, HPC, Optimization
Start Date: January 1, 2023
End Date: December 31, 2025
The project is aimed to build a transnational, scalable Federated Learning (FL) framework, which can hopefully can be scaled up according to the participating countries/units. To reify this transnational environment, the optimization of configurations of queues for HPC system is attempted. The FL learning will be used to optimize the configurations of queuing system using, but not sharing, data from supercomputers of the two participant organizations. With better configurations of queuing system, the time to solution of simulations can be reduced and thus improve the utilization of computing systems. Should the approach be successful, a ML model or a procedure that optimizes the configuration of queues that manages computing resource of a supercomputer will be obtained. Instead of ad hoc guess of configuration, a systematical way to configure queuing system will be available.
CENTRA Primary Investigators: Weicheng Huang, Research Fellow, NCHC, Taiwan; Jason Haga, Chief Senior Research Scientist, AIST, Japan
Project participants include: NCHC (Taiwan): Shun-Tai Wang, Research Scientist, Gavin Chen, Engineer, Hsiu-Mei Chou, Associated Research Fellow, Yen-Han Chiang, Assistant Research Fellow; NTOU (Taiwan): Pearl Weng, Associated Professor; AIST (Japan): Yusuke Tanimura, Senior Research Scientist, Peng Chen, Research Scientist, Truong Thao Nguyen, Research Scientist
Start Date: January 1, 2023
End Date: December 31, 2025
The project is aimed to build a transnational, scalable Federated Learning (FL) framework, which can hopefully can be scaled up according to the participating countries/units. To reify this transnational environment, the optimization of configurations of queues for HPC system is attempted. The FL learning will be used to optimize the configurations of queuing system using, but not sharing, data from supercomputers of the two participant organizations. With better configurations of queuing system, the time to solution of simulations can be reduced and thus improve the utilization of computing systems. Should the approach be successful, a ML model or a procedure that optimizes the configuration of queues that manages computing resource of a supercomputer will be obtained. Instead of ad hoc guess of configuration, a systematical way to configure queuing system will be available.
CENTRA Primary Investigators: Weicheng Huang, Research Fellow, NCHC, Taiwan; Jason Haga, Chief Senior Research Scientist, AIST, Japan
Project participants include: NCHC (Taiwan): Shun-Tai Wang, Research Scientist, Gavin Chen, Engineer, Hsiu-Mei Chou, Associated Research Fellow, Yen-Han Chiang, Assistant Research Fellow; NTOU (Taiwan): Pearl Weng, Associated Professor; AIST (Japan): Yusuke Tanimura, Senior Research Scientist, Peng Chen, Research Scientist, Truong Thao Nguyen, Research Scientist
Contact: Weicheng Huang and Jason Haga
Email: whuang (at) narlabs (dot) org (dot) tw, jh (dot) haga (at) aist (dot) go (dot) jp
Email: whuang (at) narlabs (dot) org (dot) tw, jh (dot) haga (at) aist (dot) go (dot) jp
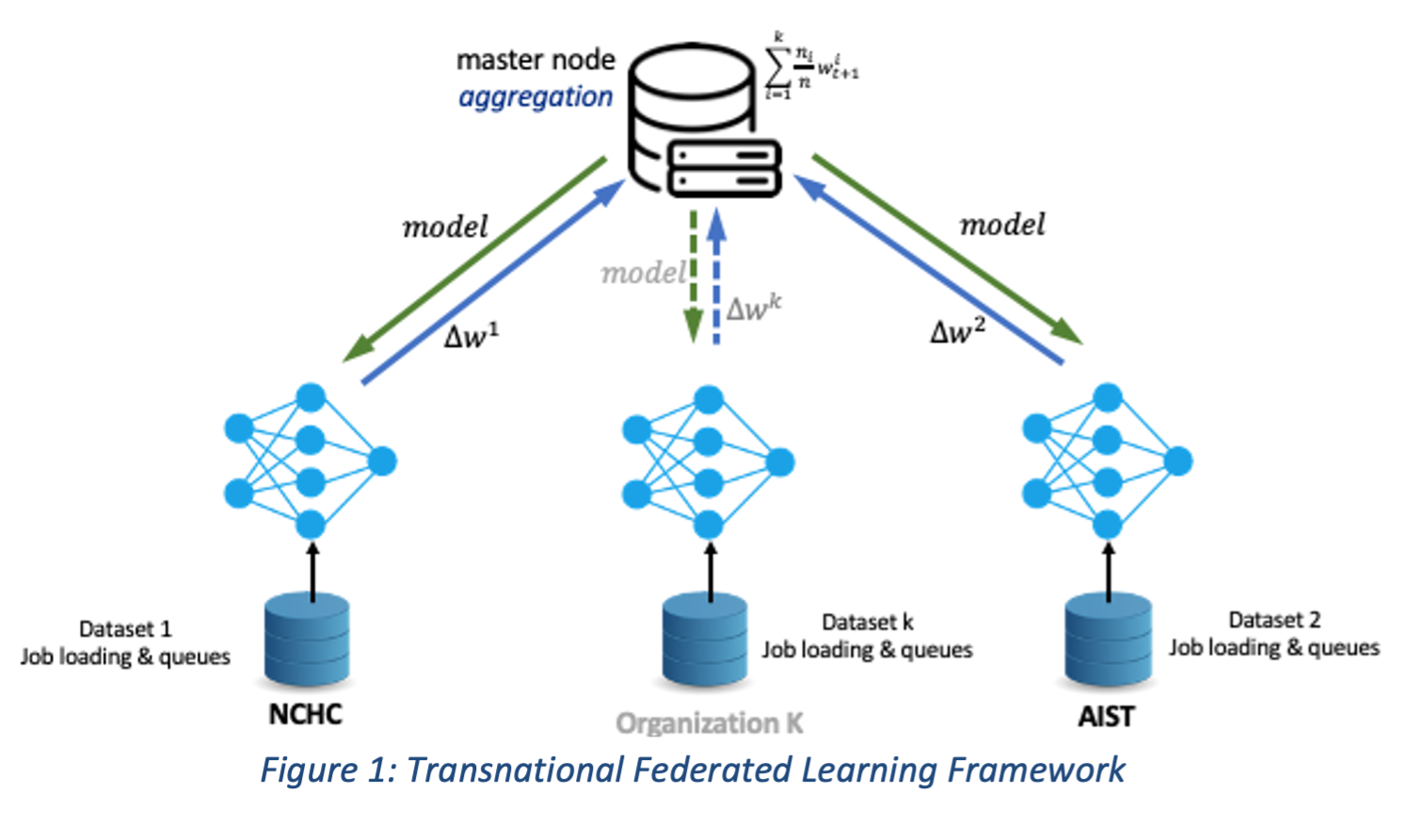
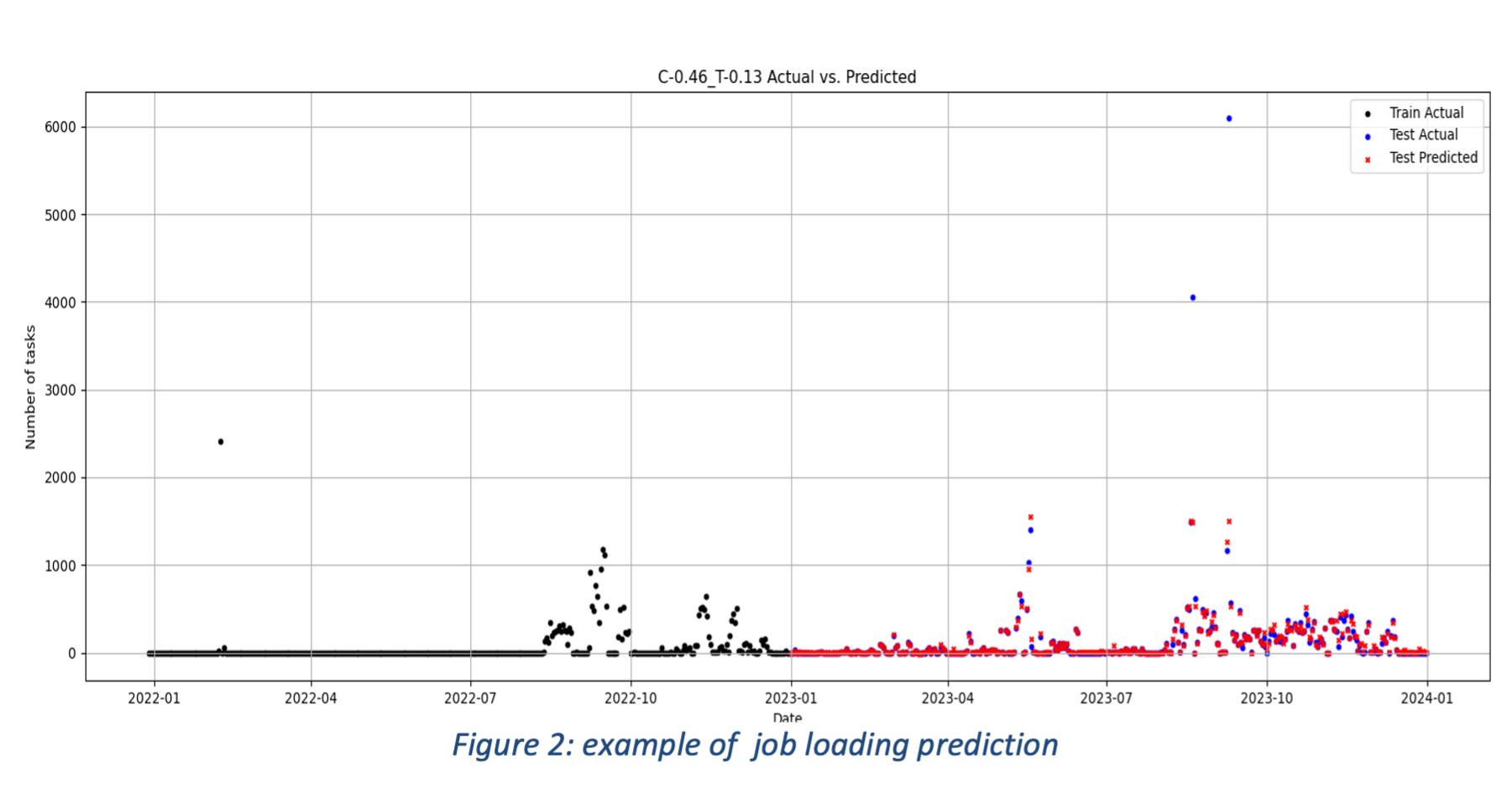
FLAG project (Federated Learning AGriculture)
Key Topics: AI, Federated Learning, Agriculture
Start Date: May 1, 2024 (Updated: February 1, 2024)
End Date: April 30, 2026
Based on the previous case study using remote sensing images to identify agricultural disaster damage in Taiwan, we further developed Federated Learning (FL) technology for agricultural disaster damage identification. Federated learning, a method that enables multiple devices to train machine learning models without sharing raw data, is particularly important in agriculture because it protects data privacy while improving the efficiency and accuracy of agricultural management. Using FL, agricultural data can be used to identify disasters, such as lodging, pests and diseases, climate change impact, and provide immediate responses and management recommendations. FL also allows model training on multiple farm devices to improve model accuracy and versatility. By building an AI model and data stores, we can create a smooth channel for sharing models and data, which will facilitate the research advancement of multinational teams. Concept of and study structure of Federated Learning Agriculture (FLAG) are illustrated as follows.
CENTRA Primary Investigators: Beth Plale, Co-director, Indiana University, Ming-Der Yang, Director, National Chung Hsing University, Ceasar Sun, Hsiu-Mei Chou, NCHC
Project participants include: Indiana University (USA): Beth Plale, Co-director; National Chung Hsing University (Taiwan): Ming-Der Yang, Director, Huiping Tsai, Cloud Tseng, Y.I. Chen; NCHC (Taiwan): Ceasar Sun, Hsiu-Mei Chou
Start Date: May 1, 2024 (Updated: February 1, 2024)
End Date: April 30, 2026
Based on the previous case study using remote sensing images to identify agricultural disaster damage in Taiwan, we further developed Federated Learning (FL) technology for agricultural disaster damage identification. Federated learning, a method that enables multiple devices to train machine learning models without sharing raw data, is particularly important in agriculture because it protects data privacy while improving the efficiency and accuracy of agricultural management. Using FL, agricultural data can be used to identify disasters, such as lodging, pests and diseases, climate change impact, and provide immediate responses and management recommendations. FL also allows model training on multiple farm devices to improve model accuracy and versatility. By building an AI model and data stores, we can create a smooth channel for sharing models and data, which will facilitate the research advancement of multinational teams. Concept of and study structure of Federated Learning Agriculture (FLAG) are illustrated as follows.
CENTRA Primary Investigators: Beth Plale, Co-director, Indiana University, Ming-Der Yang, Director, National Chung Hsing University, Ceasar Sun, Hsiu-Mei Chou, NCHC
Project participants include: Indiana University (USA): Beth Plale, Co-director; National Chung Hsing University (Taiwan): Ming-Der Yang, Director, Huiping Tsai, Cloud Tseng, Y.I. Chen; NCHC (Taiwan): Ceasar Sun, Hsiu-Mei Chou
Contact: Ming-Der Yang
Email: mdyang (dot) dragon (at) nchu (dot) edu (dot) tw
Email: mdyang (dot) dragon (at) nchu (dot) edu (dot) tw
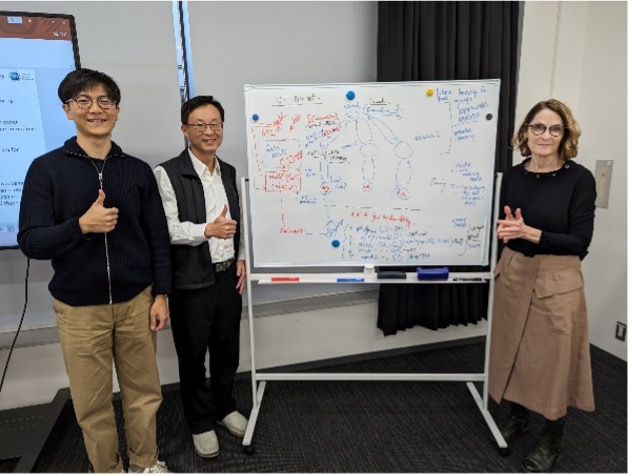
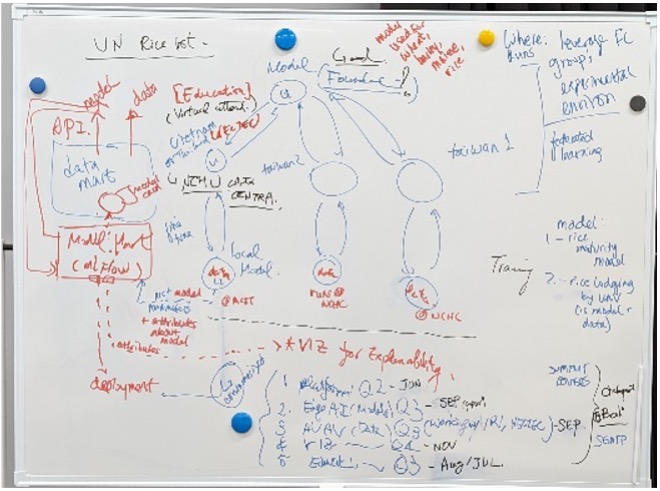
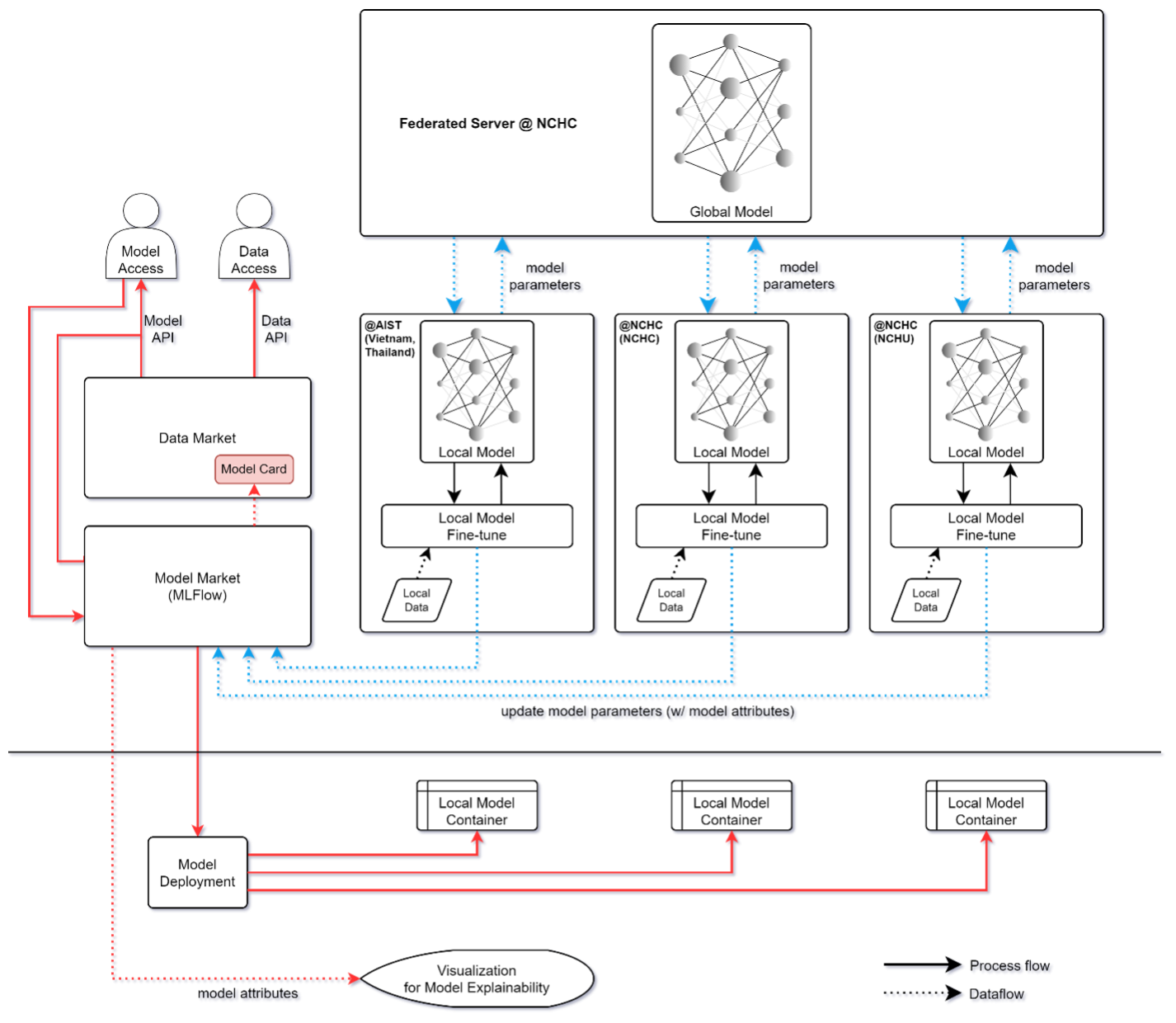
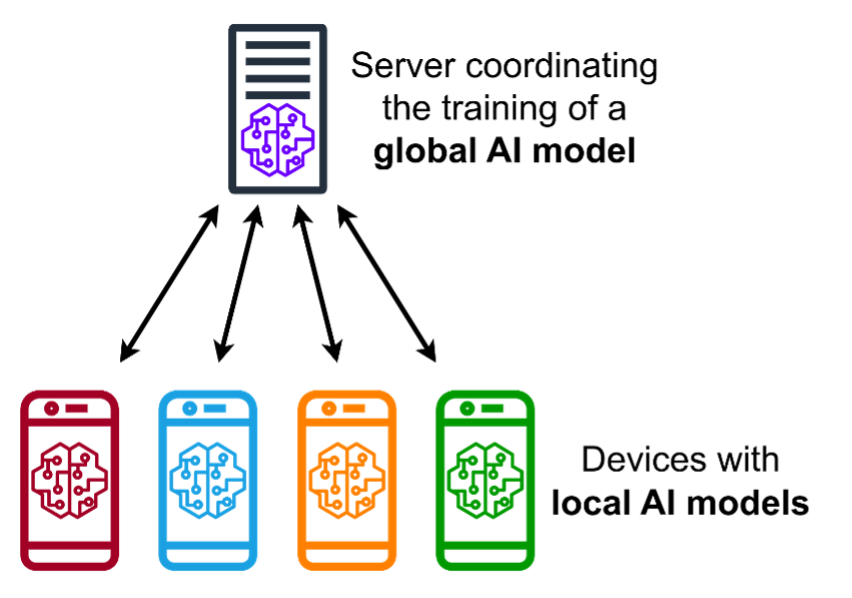
Performance and Sustainability Management in Modern Data Centers (PASM)
Key Topics: Performance, Sustainability, Modern Infrastructures
Start Date: May 9, 2024
The “Performance and Sustainability Management in Modern Data Centers” project, led by Ricardo Macedo (INESC TEC), focuses on designing new computer systems and techniques to meet the performance, reliability, and environmental sustainability of modern data centers. The project is guided by three primary goals. First, it seeks to harness cutting-edge technologies present in modern data centers --- such as persistent memory, CXL, resource disaggregation, among others --- to design the next generation of storage, memory, and network systems. These advancements aim to improve the performance of large-scale applications and workloads, such as LLM training and inference, scientific simulations, and data-centric applications. Second, the project explores new software-driven approaches to balance the application performance and the energy consumption and carbon footprint of data center infrastructures, using dynamic frequency scaling, thermal management and cooling system control, and carbon-aware scheduling. Finally, the project aims to bridge the gap between research and real-world application by creating accessible, well-tested solutions tailored to the challenges faced in production environments, promoting adoption by academia and industry.
CENTRA Primary Investigators: Ricardo Macedo, Researcher, INESC TEC, Portugal
Project participants include: INESC TEC (Portugal): Ricardo Macedo, Researcher, João Paulo, Senior Researcher, Cláudia Brito, Researcher, José Pedro Peixoto, PhD student; AIST (Japan): Jason Haga, Chief Senior Research Scientist, Yusuke Tanimura, Senior Researcher; FIU (USA): Raju Rangaswami, Eminent Scholar Chaired Professor, Janki Bhimani, Assistant Professor, Yanzhao Wu, Assistant Professor, Jason Liu, Endowed Computer Science Professor & Director
Start Date: May 9, 2024
The “Performance and Sustainability Management in Modern Data Centers” project, led by Ricardo Macedo (INESC TEC), focuses on designing new computer systems and techniques to meet the performance, reliability, and environmental sustainability of modern data centers. The project is guided by three primary goals. First, it seeks to harness cutting-edge technologies present in modern data centers --- such as persistent memory, CXL, resource disaggregation, among others --- to design the next generation of storage, memory, and network systems. These advancements aim to improve the performance of large-scale applications and workloads, such as LLM training and inference, scientific simulations, and data-centric applications. Second, the project explores new software-driven approaches to balance the application performance and the energy consumption and carbon footprint of data center infrastructures, using dynamic frequency scaling, thermal management and cooling system control, and carbon-aware scheduling. Finally, the project aims to bridge the gap between research and real-world application by creating accessible, well-tested solutions tailored to the challenges faced in production environments, promoting adoption by academia and industry.
CENTRA Primary Investigators: Ricardo Macedo, Researcher, INESC TEC, Portugal
Project participants include: INESC TEC (Portugal): Ricardo Macedo, Researcher, João Paulo, Senior Researcher, Cláudia Brito, Researcher, José Pedro Peixoto, PhD student; AIST (Japan): Jason Haga, Chief Senior Research Scientist, Yusuke Tanimura, Senior Researcher; FIU (USA): Raju Rangaswami, Eminent Scholar Chaired Professor, Janki Bhimani, Assistant Professor, Yanzhao Wu, Assistant Professor, Jason Liu, Endowed Computer Science Professor & Director
Contact: Ricardo Macedo
Email: ricardo (dot) g (dot) macedo (at) inesctec (dot) pt
Email: ricardo (dot) g (dot) macedo (at) inesctec (dot) pt
The Visualization Alliance
The "Visualization Alliance" project, led by Jason Leigh (U. Hawaii at Manoa), considers research questions that arise when building and managing a cyberinfrastructure for visualization of data and cooperative work across geographically distributed sites. Each CENTRA site has a visualization wall, and associated computers and storage resources to execute a tool (SAGE2) that allows different walls to be shared and synchronized, and ancillary data analytics and simulations. SAGE2 has been developed by J. Leigh's group at Hawaii at Manoa and the Electronic Visualization Laboratory of the U. Illinois at Chicago. Current sites include institutions in the USA (U. Hawaii at Manoa and U. Florida), Taiwan (NCHC) and Japan (AIST and Osaka U.). The domain contexts for the research questions addressed by this project includes decision support and outreach in energy, coral reef, water, and disaster management. The middleware research context includes the leveraging of Software Defined Networks to provide network QoS, security and integration for critical decision support. Additional IT issues considered include the use of persistent data identifiers to better enable the joining of data sets to be visualized, support for merging big-data analysis with machine learning, and the development of a tool for distributed cooperative computer-enhanced brainstorming.
Joint project participants with IT for Natural Disaster Management group: U of Hawaii at Manoa (USA): Jason Leigh, Dylan Kobayashi; AIST (Japan): Jason Haga; NCHC (Taiwan): Whey-Fone Tsai; U of Hawaii Systems (USA): Bill Chang; UIC (USA): Maxine Brown; Osaka University (Japan): Yoshiyuki Kido
Joint project participants with IT for Natural Disaster Management group: U of Hawaii at Manoa (USA): Jason Leigh, Dylan Kobayashi; AIST (Japan): Jason Haga; NCHC (Taiwan): Whey-Fone Tsai; U of Hawaii Systems (USA): Bill Chang; UIC (USA): Maxine Brown; Osaka University (Japan): Yoshiyuki Kido
Contact: Jason Leigh
Email: leighj (at) hawaii (dot) edu
Email: leighj (at) hawaii (dot) edu
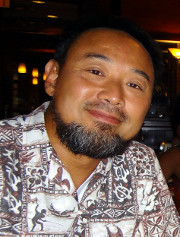
SDN-IP Peering for IOT Data Transmission
The "SDN-IP Peering for IOT Data Transmission" project, led by Te-Lung Liu (NCHC, Taiwan) and Shinji Shimojo (NICT, Japan) studies architectures and mechanisms to federate IP Networks with software-defined IP networking for resilient and effective infrastructure for disaster mitigation and smart city applications. In order to meet the needs of inter-domain transmissions, we would like to setup a adaptive network transmission testbed based on SDN-IP with efficiency, elasticity, security, and convenience. We exploit the L3 routing mechanism of SDN-IP for inter-domain communication for interconnecting several research sites. For end users, a local router is installed for distributing packets between SDN-IP and legacy Internet. Therefore, client hosts could transmit the packets without IP address modification. With their original IP addresses, the researchers could not only access legacy Internet but also exchange information over the SDN-IP backbone.
Project participants include: NICT (Japan): Shinji Shimojo, Naomi Terada, Yoshihiko Kanaumi, Eiji Kawai; NCHC (Taiwan): Te-Lung Liu, Jen-Wei Hu, Grace Hui-Lan Lee
Project participants include: NICT (Japan): Shinji Shimojo, Naomi Terada, Yoshihiko Kanaumi, Eiji Kawai; NCHC (Taiwan): Te-Lung Liu, Jen-Wei Hu, Grace Hui-Lan Lee
Contact: Te-Lung Liu
Email: tlliu (at) nchc (dot) narl (dot) org (dot) tw
Email: tlliu (at) nchc (dot) narl (dot) org (dot) tw
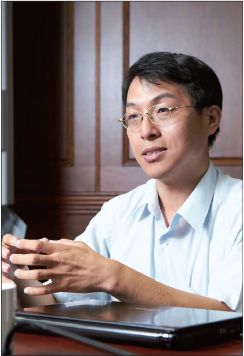
Efficient and Secure Data Management for HPC and Cloud Computing
The "Efficient and Secure Data Management for HPC and Cloud Computing" project, led by João Paulo (INESC TEC), aims at researching novel data management solutions for Cloud and HPC distributed environments. These solutions must be efficient, secure, and practical by: 1) alleviating any storage or processing performance bottlenecks identified for data-centric applications deployed on HPC and Cloud services; 2) following a privacy-by-design approach when storing and processing sensitive data on third-party infrastructures; and 3) re-using stable and industry-adopted storage systems, databases and analytical platforms.
Currently, the project contemplates solutions for file systems, SQL and NoSQL databases, as well as analytical and AI platforms. The ambition of this project is to leverage collaboration between CENTRA partners, as well as other researchers, that are interested in these research areas and/or have good use-cases for validating the project’s outcomes.
Project team members include: INESC TEC (Portugal): João Paulo, Ricardo Macedo, Tânia Esteves, Cláudia Brito, and Cláudia Correia; AIST (Japan): Yusuke Tanimura, Jason Haga; KISTI (S. Korea); Woojin Seok.
Currently, the project contemplates solutions for file systems, SQL and NoSQL databases, as well as analytical and AI platforms. The ambition of this project is to leverage collaboration between CENTRA partners, as well as other researchers, that are interested in these research areas and/or have good use-cases for validating the project’s outcomes.
Project team members include: INESC TEC (Portugal): João Paulo, Ricardo Macedo, Tânia Esteves, Cláudia Brito, and Cláudia Correia; AIST (Japan): Yusuke Tanimura, Jason Haga; KISTI (S. Korea); Woojin Seok.
Contact: João Paulo
Email: jtpaulo (at) inesctec (dot) pt
Email: jtpaulo (at) inesctec (dot) pt
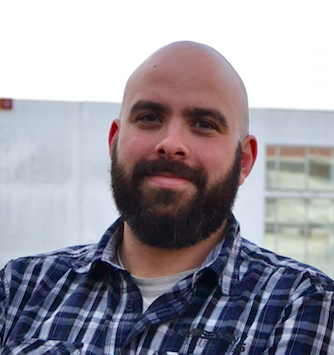
Persistent Identifier (PID) Kernel Information
The "Persistent Identifier (PID) Kernel Information" project, led by Beth Plale (Indiana University), considers the problem of enabling persistent IDs (PIDs) in environmental and biodiversity data collections, using a Robust Persistent IDentifier (RPID) testbed to evaluate PID services. In part through engagement with the Research Data Alliance, the project is defining a minimal set of metadata (called PID kernel information) that travels with PIDs and can be processed at Internet speeds by data discovery services. The novelty of the work emerges when a small amount of data provenance is included amongst the kernel information. This provenance enables a universal provenance for research data, where all data objects created as a result of scientific research have data provenance associated with them, moving us to a world where assessments of fitness and trust for secondary use of research data can be done with far higher degrees of success than can be achieved today.
Contact: Beth Plale
Email: plale (at) indiana (dot) edu
Email: plale (at) indiana (dot) edu
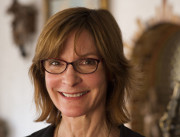
IT for Natural Disaster Management
The "IT for Natural Disaster Management" project, led by Jason Haga (National Institute for Advanced Industrial Science and Technology (AST)), considers the use of distributed immersive visualization and analysis systems (developed under the Visualization Alliance) to support activities needed to respond to river-related disasters. This research uses an interdisciplinary effort to tackle a variety of areas including the development of novel interaction modalities for grappling with big datasets, heterogeneous data integration through layered/linked visualizations, userinterface/user-experience research to test and improve the usability of applications in immersive visualization environments, and infrastructure integration through flexible (SDN) networks to provide a level of resilience to the system.
Joint project participants with Visualization Alliance: AIST (Japan): Jason Haga; U of Hawaii at Manoa (USA): Jason Leigh, Dylan Kobayashi; NCHC (Taiwan): Whey-Fone Tsai; U of Hawaii Systems (USA): Bill Chang; UIC (USA): Maxine Brown; Osaka University (Japan): Yoshiyuki Kido
Joint project participants with Visualization Alliance: AIST (Japan): Jason Haga; U of Hawaii at Manoa (USA): Jason Leigh, Dylan Kobayashi; NCHC (Taiwan): Whey-Fone Tsai; U of Hawaii Systems (USA): Bill Chang; UIC (USA): Maxine Brown; Osaka University (Japan): Yoshiyuki Kido
Contact: Jason Haga
Email: jh (dot) haga (at) aist (dot) go (dot) jp
Email: jh (dot) haga (at) aist (dot) go (dot) jp
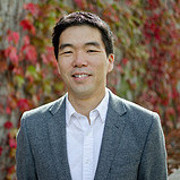
Dynamically Aggregating Smart Community Sensors, Edge and Cloud Resources with Overlay VPNs
The "Dynamically Aggregating Smart Community Sensors, Edge and Cloud Resources with Overlay VPNs" project, led by Renato Figueiredo (University of Florida), researches techniques to dynamically connect groups of distributed devices - Internet-of-Things (IoT) sensors/actuators, edge, and cloud computing resources - through a virtual private network (VPN). At the network layer, the VPN is built upon P2P tunnels across the Internet, automatically handling NAT and firewall traversal, exposes a virtual network abstraction to software-defined switches, and is transparent to middleware and applications. The project also researches techniques at the middleware layer (for the dynamic instantiation of stream processing workflows across edge/cloud resources) and at the application layer. Target applications include smart/connected community scenarios where users need to collaborate or cooperate in dynamic, time-limited ways and/or form ad-hoc groups, volunteering resources to help respond to emergencies.
Overview of the project and recent development is available in this video webinar recording (October 10th, 2017)
Project team members include: UF (USA): Renato Figueiredo, Kensworth Subratie, Saumitra Aditya, Vahid Daneshmand; NICT (Japan): Eiji Kawai, Naomi Terada, Hiroaki Yamanaka; Osaka University (Japan): Susumu Date, Yoshiuki Kido, Takuya Yamada; AIST (Japan): Ryuousei Takano; NAIST (Japan): Kohei Ichikawa; Seoul National University (S. Korea): Hyuk-Jae Lee; NII (Japan): Jingtao Sun, Atsuko Takefusa, Tomoya Tanjo
Overview of the project and recent development is available in this video webinar recording (October 10th, 2017)
Project team members include: UF (USA): Renato Figueiredo, Kensworth Subratie, Saumitra Aditya, Vahid Daneshmand; NICT (Japan): Eiji Kawai, Naomi Terada, Hiroaki Yamanaka; Osaka University (Japan): Susumu Date, Yoshiuki Kido, Takuya Yamada; AIST (Japan): Ryuousei Takano; NAIST (Japan): Kohei Ichikawa; Seoul National University (S. Korea): Hyuk-Jae Lee; NII (Japan): Jingtao Sun, Atsuko Takefusa, Tomoya Tanjo
Contact: Renato Figueiredo
Email: renato (at) acis (dot) ufl (dot) edu
Email: renato (at) acis (dot) ufl (dot) edu
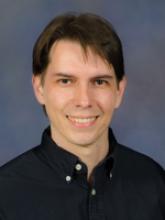
Collaborative Lifemapper
The "Collaborative Lifemapper" project led by James Beach and Aimee Stewart (U. Kansas) is pursuing the use of species distribution and macroecological modeling for biogeographic, phylogenetic, and biodiversity analyses of plant and animal species in North America and Asia. The effort utilizes unique Lifemapper installations for CENTRA sites using researcher-specified or online species occurrence data along with observed climate and predicted climate data based on IPCC scenarios, as well as topographic and soils data to explain the ecological and evolutionary contributions to observed spatial patterns of species diversity. The Lifemapper platform is designed to work with Rocks clusters using the Rocks roll packaging system allowing it to be consistently deployed in different computational environments (Rocks software is developed and maintained by SDSC CENTRA researcher Phil Papadopoulos and his team). Rolls contain software packages as RPMs which configure the platform for particular virtual or physical hardware. The platform is deployed in the USA using virtual clusters in the XSEDE program's resource 'Comet' enabling agile and rapid scaling to match computational load. It is also deployed at the Nat. Center for HPC, NARLabs, Taiwan on OpenStack with Taiwanese data. In collaboration with the CENTRA Visualization Alliance this project is developing a compatible front-end Lifemapper application for the SAGE2 visualization wall for biogeographical pattern discovery and cooperative work across CENTRA countries.
Project team members also include: U. Kansas (USA): Aimee Stewart, James Beach; NCHC (Taiwan): Hsiu-Mei Chou; INESC TEC (Portugal): João R. Silva; UCSD (USA): Nadya Williams, UF (USA): Matthew Collins
Project team members also include: U. Kansas (USA): Aimee Stewart, James Beach; NCHC (Taiwan): Hsiu-Mei Chou; INESC TEC (Portugal): João R. Silva; UCSD (USA): Nadya Williams, UF (USA): Matthew Collins
Contact: James Beach
Email: beach (at) ku (dot) edu
Email: beach (at) ku (dot) edu
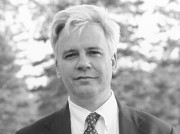
AUAV Applications for Smart Agriculture
The “AUAV applications for smart agriculture” project, co-led by Ming-Der Yang, Hui Ping Tsai (NCHU, Taiwan) and Christopher Stewart (OSU), considers the use of unmanned aerial vehicle (UAV) for agriculture applications with integrating two major research directions together, edge computing for image processing with object recognition and autonomous UAV technique development. At the moment, rice in Taiwan and corn in Ohio USA are two focusing crops that have been observed. Promising results of over 80% accuracy in object recognition by edge computing and a fully autonomous UAV system has been developed from the efforts of both side researchers.
Project team members also include: NCHU (Taiwan): Ming-Der Yang, Yu-Chun Hsu, Hsin-Hung (Cloud) Tseng; NCHC (Taiwan): Fang-Pang Lin; University of Kentucky (USA): Corey Baker; UC Davis (USA): S. Felix Wu
Project team members also include: NCHU (Taiwan): Ming-Der Yang, Yu-Chun Hsu, Hsin-Hung (Cloud) Tseng; NCHC (Taiwan): Fang-Pang Lin; University of Kentucky (USA): Corey Baker; UC Davis (USA): S. Felix Wu
Contact: Hui Ping Tsai and Christopher Stewart
Email: huiping.tsai (at) gmail (dot) com, cstewart (at) cse (dot) ohio-state (dot) edu
Email: huiping.tsai (at) gmail (dot) com, cstewart (at) cse (dot) ohio-state (dot) edu
AirBox
The "AirBox" project, led by Ling-Jyh Chen (Academia Sinica, Taiwan), looks at the problem of designing and deploying affordable accurate sensors worldwide to measure particulate matter (PM2.5), aggregating data from these sensors, opening data to access by the public and analyzing the data to explain causes and detect sources of pollution across multiple countries.
Project collaborators also include: Argonne National Laboratory and Array of Things project (USA): Charlie Catlett; US Ignite (USA): Glenn Ricart; UCSD (USA): Shava Smallen; UC Davis (USA): S. Felix Wu; NCHC (Taiwan): Fang-Pang Lin; ASEAN-IVO project members (Malaysia, Myanmar, Philippines, Singapore, Thailand, Vietnam); NICT (Japan): Shinji Shimojo
Project collaborators also include: Argonne National Laboratory and Array of Things project (USA): Charlie Catlett; US Ignite (USA): Glenn Ricart; UCSD (USA): Shava Smallen; UC Davis (USA): S. Felix Wu; NCHC (Taiwan): Fang-Pang Lin; ASEAN-IVO project members (Malaysia, Myanmar, Philippines, Singapore, Thailand, Vietnam); NICT (Japan): Shinji Shimojo
Contact: Ling-Jyh Chen
Email: cclljj (at) gmail (dot) com
Email: cclljj (at) gmail (dot) com
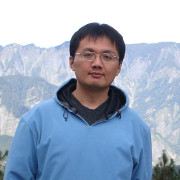